Organizations are transitioning from generic solutions to hyper-customized intelligence, seeking AI models designed to address their unique challenges and propel them toward strategic objectives. This demand has propelled AI fine-tuning to the forefront of AI development – a strategic technique that transforms pre-trained models into bespoke business partners, meticulously aligned with an organization's own data and aspirations.
Fine-tuning can be complex, requiring expertise in selecting the right pre-trained model, managing data quality, and avoiding overfitting – that's where Agente's AI engineers can ensure a smooth and successful process.
We've witnessed this shift firsthand through our work on various AI projects. We've seen how fine-tuning empowers businesses to unlock the true potential of AI, and in this article, we'll share our insights to help you leverage this powerful technique for your own success. Let's break down the process of fine-tuning AI, its benefits, and how to apply it to extract the most value for your strategic goals.
Benefits of Fine-Tuning in AI & What Is It?
Fine-tuning AI is the meticulous process of adjusting a pre-trained model's internal parameters to align with your specific data and desired outputs. Think of the model as a complex algorithm, a system of interconnected mathematical functions. Fine-tuning strengthens the functions relevant to your objectives while carefully pruning those that deviate, reshaping the algorithm into a dedicated engine optimized for your unique tasks.
By engaging in AI fine-tuning, organizations reap a multitude of benefits:
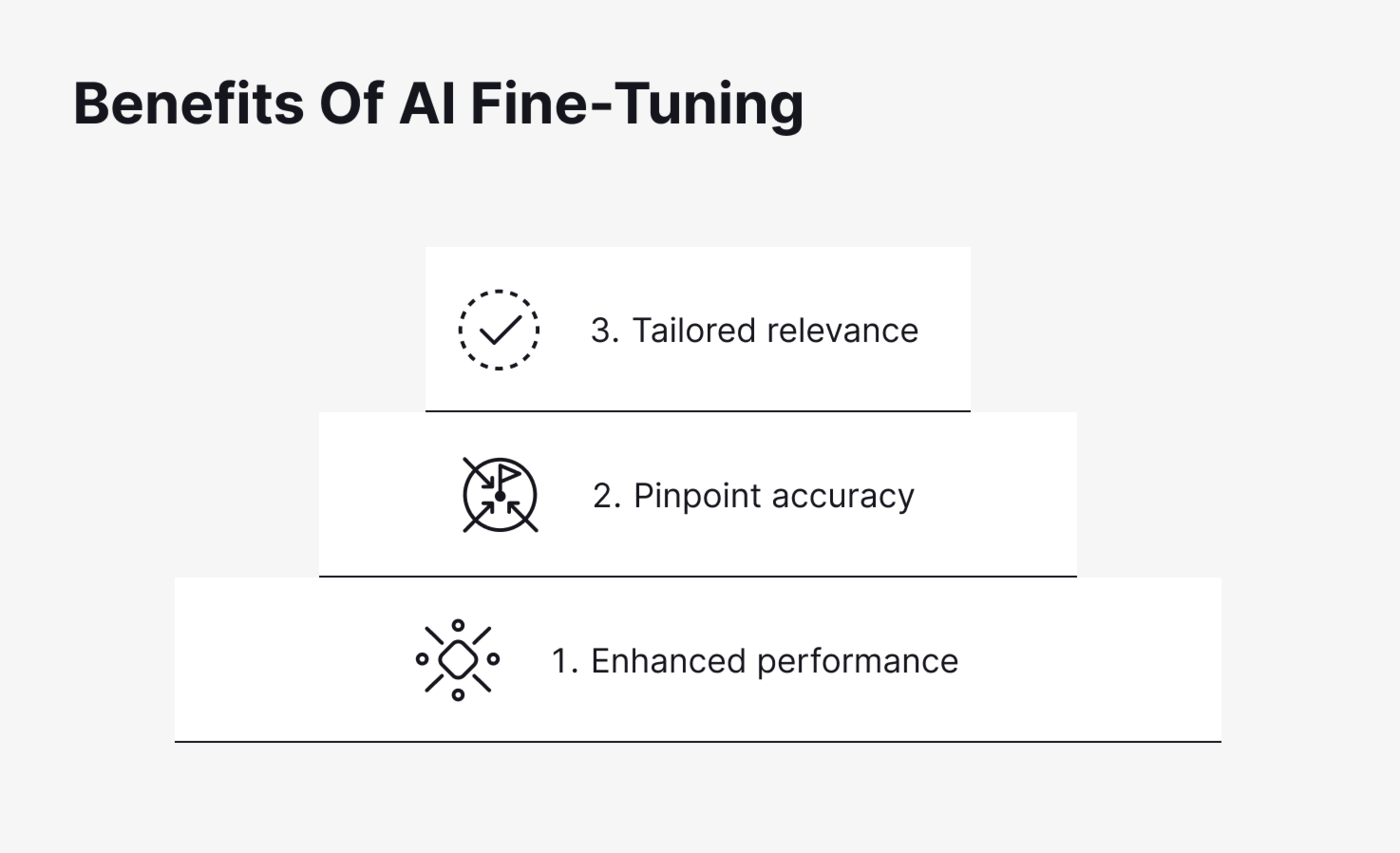
- Enhanced performance. The model leverages existing knowledge from the pre-trained base, requiring less training data and achieving superior accuracy on specific tasks.
- Pinpoint accuracy. The focus on a specific domain reduces noise and irrelevant information, leading to more refined and reliable results.
- Tailored relevance. The model becomes adept at handling the language and complexities of your chosen domain, generating outputs that directly address your unique needs.
Ultimately, AI fine-tuning for business empowers organizations to transcend the limitations of generic AI and get the intelligence that understands their language, anticipates their needs, and seamlessly integrates into their existing processes. With this new type of relationship, a business can unlock a new era of possibilities, optimize workflows, generate deep data insights, and drive unprecedented strategic advantage.
The Process of Fine-Tuning an AI Model
But navigating this nuanced process requires careful planning and execution. While the specifics may differ depending on the chosen platform and model, the core steps remain a roadmap for success:
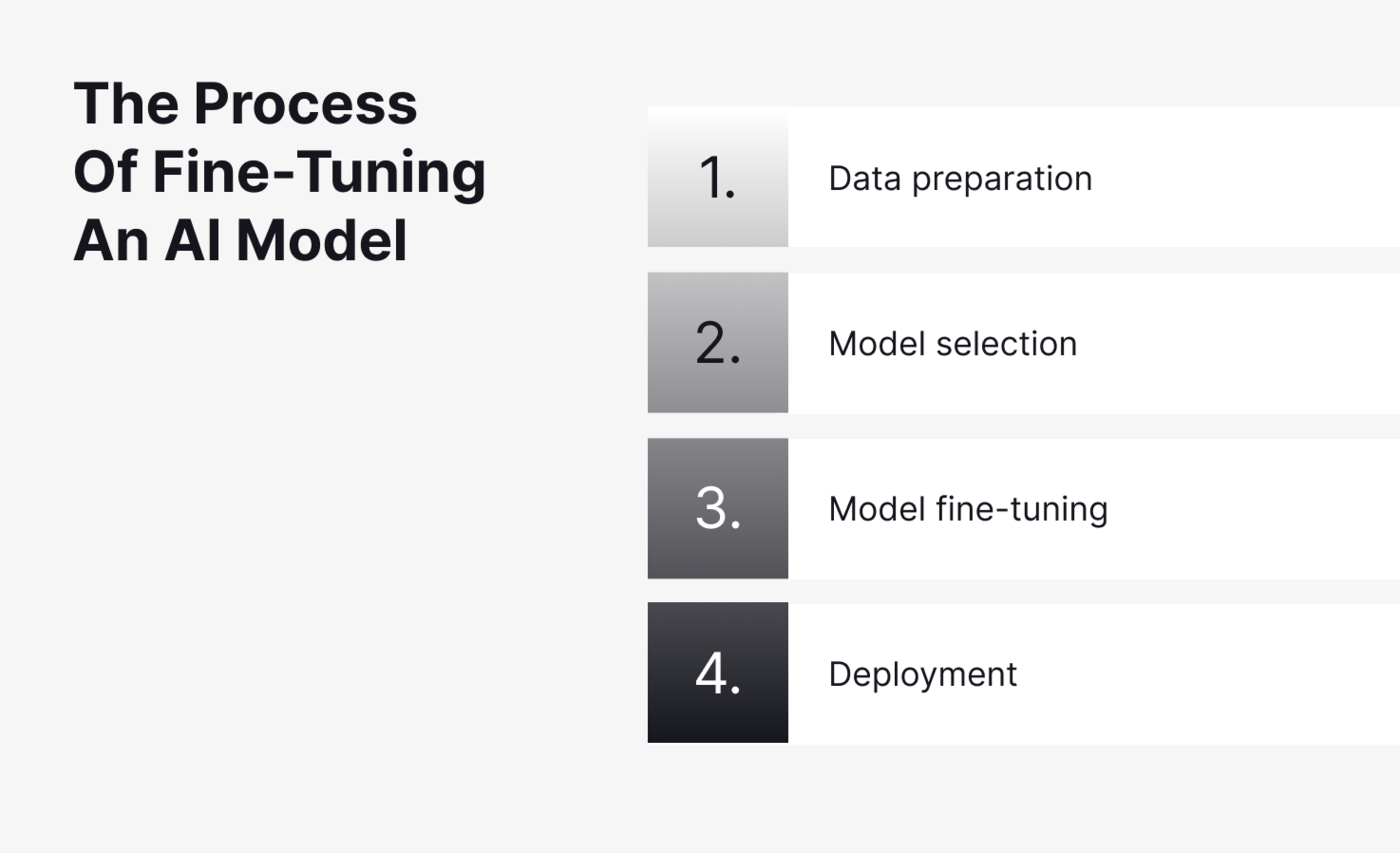
Data preparation
Before embarking on the fine-tuning journey, prepare your data – the fuel that will power your AI engine. This crucial stage consists of four key steps:
- Data collection. Gather high-quality data relevant to your target task or domain. Ensure it accurately reflects real-world scenarios the model will encounter.
- Data cleaning. Scrutinize your data for errors, inconsistencies, and duplicates. These can significantly skew the model's learning process and compromise its accuracy.
- Data preprocessing. Standardize your data format and structure to align with the chosen platform and model requirements. This may involve normalization, tokenization, or feature engineering.
- Data labeling. For tasks requiring supervised learning, assign accurate labels to data points, guiding the model in its understanding of desired outputs.
Model selection
Choosing the right pre-trained model is critical for optimal performance. Analyze your needs through these lenses:
- Identify task requirements. What specific task or domain will the model be used for? A language model like GPT-3 wouldn't excel at image recognition, while a computer vision model wouldn't be ideal for text analysis.
- Consider data format. Ensure the model's input format aligns with your prepared data. Text analysis models won't understand image data, and vice versa.
- Evaluate computational resources. Consider your available computational resources and choose a model compatible with your infrastructure. Complex models may require significant processing power.
- Explore pre-trained models. Research existing pre-trained models with proven performance in similar tasks or domains. Benchmarking results can assist in making an informed decision.
Model fine-tuning
This is where the magic happens – where your data transforms the pre-trained model into a bespoke solution. Engage in meticulous fine-tuning through these crucial steps:
- Hyperparameter optimization. Adjust the internal parameters of the model to optimize its learning process for your specific data and objectives. Think of it as fine-tuning a telescope to bring your desired target into focus.
- Loss function selection. Choose an appropriate loss function to measure the discrepancy between the model's outputs and desired results. This guides the AI model's adjustments in subsequent training iterations.
- Evaluation metrics. Establish clear metrics to assess the model's performance throughout training and deployment. Common metrics include accuracy, precision, recall, and F1 score.
- Bias mitigation. Be mindful of potential biases within your data or the pre-trained model. Implement techniques like data augmentation and fairness algorithms to counteract biases and ensure ethical AI development.
Deployment
With a meticulously fine-tuned model in hand, it's time to integrate it into your workflow for real-world impact. Consider these crucial aspects:
- Deployment environment. Choose a platform to host the model that aligns with your desired scalability, security, and operational needs. Cloud platforms offer flexibility and accessibility.
- Monitoring and feedback. Continuously monitor the model's performance in real-world scenarios. Gather user feedback and utilize it to further refine the model through ongoing training cycles.
By following these steps and remaining diligent throughout the process, you can unlock the true potential of fine-tuning AI models. Witness your data transform into the fuel that propels your bespoke AI partner towards exceptional performance and drives real-world success for your organization.
Fine-Tuning with ChatGPT
While pre-trained AI models like ChatGPT offer immense potential, true business transformation is all about tailoring them to your specific needs. This is where fine-tuning in AI takes center stage – the potent technique that imbues ChatGPT with the knowledge of your organization, transforming it into a bespoke partner driving success.
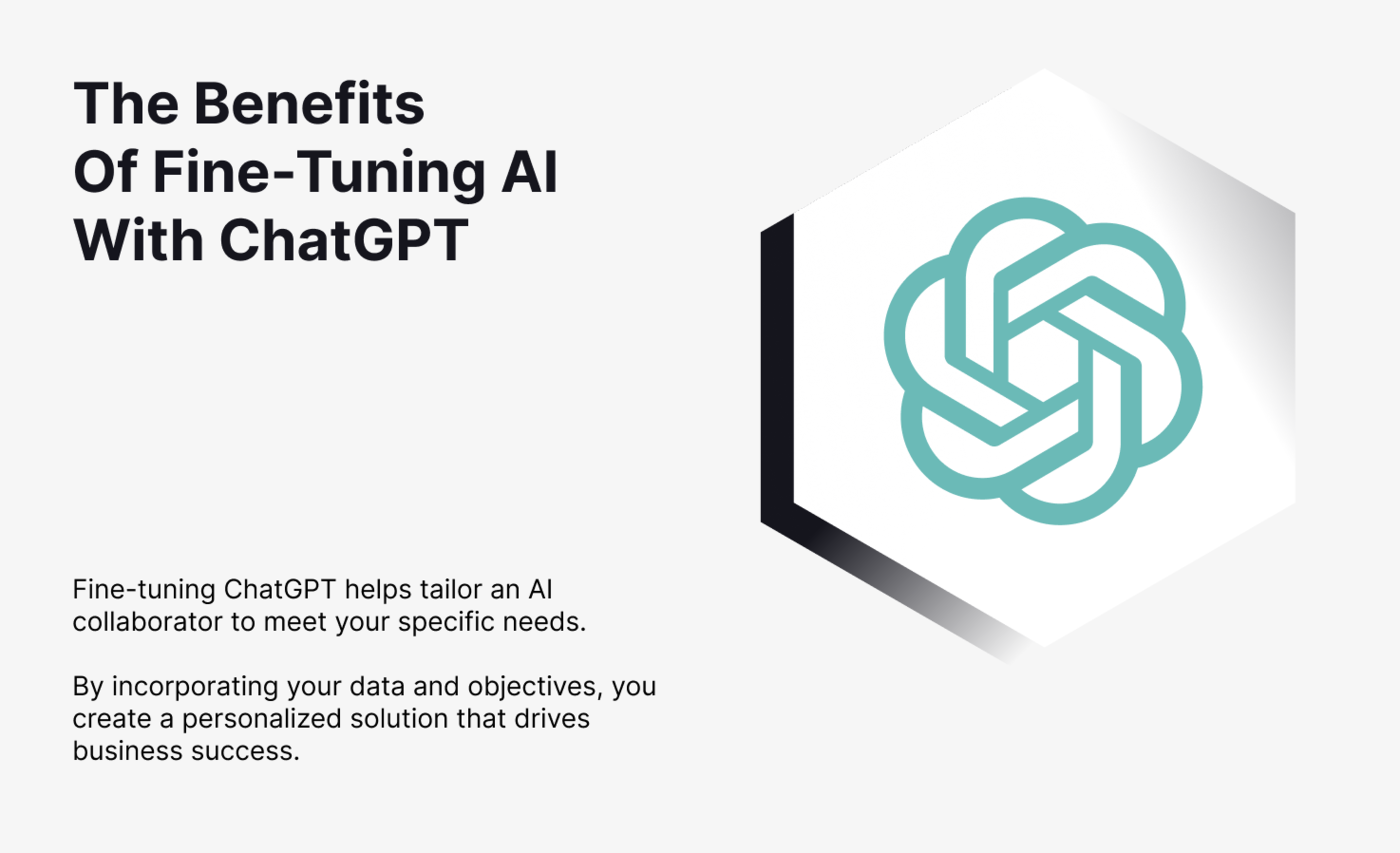
Here’s a short guide on how ChatGPT fine-tuning can be utilized to boost operational efficiency.
- Target. Decide what you want your fine-tuned ChatGPT to do (e.g., write marketing copy, summarize reports);
- Prep your data. Gather high-quality, relevant data in a format compatible with ChatGPT. Label data if needed;
- OpenAI & model. Sign up for OpenAI and choose a ChatGPT model suited to your task;
- Fine-tuning. Upload data, configure training, and track progress through OpenAI's tools;
- Evaluation & deployment. Test your fine-tuned ChatGPT, deploy it for real use, and monitor its performance;
- Bonus. Watch out for data biases and ensure security/privacy compliance.
By following these steps, you can leverage your organization's data for fine-tuning ChatGPT to craft a bespoke partner that drives tangible results.
Agente Case Studies in AI Model Development
At Agente, we elevate AI software development beyond generic solutions. Our deep expertise in fine-tuning AI models allows us to craft solutions that tackle your specific challenges head-on. We don't merely utilize AI; we make sure to tailor it to match your strategy, propelling your business toward top-tier performance.
Here are just a few examples of our approach to AI fine-tuning in business use:
Headpix: from selfie to headshot
Headpix, powered by Agente AI expertise, is an app that transforms casual selfies into polished, professional headshots in seconds. We fine-tuned an AI model to analyze facial features, lighting, and background details, then applied subtle enhancements that allow the software to create a professional image without compromising authenticity. Headpix empowers professionals of any field to project a polished image with minimal effort, redefining the landscape of online presentations and personal branding.
The AI chat assistant: your copywriting concierge
AI chat assistant was built to spare users from juggling multiple tools for translation, copywriting, and basic editing. It simplifies text-related workflows by seamlessly integrating these functionalities into a single, dynamic automation platform. Powered by a carefully fine-tuned language model, our assistant understands your instructions, translates languages with nuanced accuracy, generates email drafts tailored to your tone and objectives, and even polishes pre-written text for greater clarity and impact.
Mobile image enhancer: restore and upscale with AI
A mobile app, using AI models, allows for capturing that perfect moment even with less-than-ideal lighting or resolution. AI image enhancer utilizes sophisticated AI fine-tuning techniques to analyze and improve your photos. It can erase backgrounds, sharpen blurry details, or simply upscale a low-resolution image. With the help of advanced technology, users can preserve precious memories, enhance social media posts, or even bring old photographs back to life.
Bottom Line
However, mastering this intricate process alone is unnecessary. Agente steps in as your trusted AI partner, a team of seasoned professionals equipped to guide you through every step of the fine-tuning journey. We provide the expertise, the tools, and the know-how to transform your data into an AI masterpiece that orchestrates your business success.